When the discovery is lit, Virtual labs open up opportunities for machine learning to understand promising classes of objects. Thomas Edison tried hundreds of things and failed thousands of times before he discovered that a filament of yellow cotton burned long and brightly inside a light bulb.
Trials were often time-consuming (Edison’s team spent 14 months) and expensive (a winning team cost about $850,000 in today’s money).
Costs and time are greatly increased when designing products that will replace modern electronics and computers.
To make the discovery of quantum applications possible, researchers are turning to detailed databases as virtual laboratories. A new database of inorganic materials developed by researchers at the Pacific Northwest National Laboratory (PNNL) provides a way to identify new materials that could make appliances more powerful than Edison’s light bulb.
Passing Edisonian trial and error
“We want to understand a general class of things that have the same crystal structure, but different properties depending on how they are combined and grown,” said scientist Tim Pope. This class of material, known as transition metal dichalcogenides (TMDs), has thousands of potential combinations, each of which requires a long reaction to grow flake-sized flakes of material.
Doing the activity is just the first step to understanding what it can do. According to PNNL computer scientist Micah Prange, each flake is “really small, it’s really soft”, and the quantum features will only appear when born at high temperatures. In fact, “the entire research process can fit into every snowflake”.
Despite the difficulties of creating and measuring them, each combination promises to improve electronics, batteries, pollution control and quantum computing devices.
Prange said you can think of the flakes as “sophisticated graphene with rich phenomenology and practical possibilities.” Strong, lightweight and flexible, graphene is known as the material of the future, used in everything from space to wearable electronics.
“The different characteristics of the class of this material mean that, if we understand them well, it is possible to choose one of the links for the property that is needed and specifically linked to the real use,” Pope said, “but it is even a complete new application”.
The development of quantum objects of the future
The database was launched with the PNNL Chemical Dynamics Initiative, an effort to use PNNL’s strengths in data science to fill the knowledge gaps left by challenging measurement and experimental limitations.
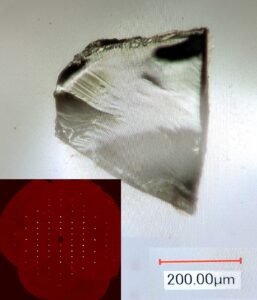
These elements are quantum different from 38 transition metals, such as tungsten or vanadium, and three elements from the sulfur family. They can be grown in three different crystal forms, meaning there are thousands of possibilities, all with different properties.
Using a model called density functional theory, the researchers calculated 672 unique structures with a total of 50,337 individual atomic configurations. Before this research, there were less than 40 structures studied, with only a rough understanding of their properties.
“Types can determine the numerical order of how atoms are arranged,” Prange said. “From this, you can tell if the material will conduct electricity or reflect or how hard it will be and the material will be compressed or bent.”
Using the database, PNNL researchers showed significant differences in electrical and magnetic behavior between different suits. Importantly, the researchers also discovered other processes through transition metals, including a new understanding of transition metal chemistry at the quantum level.
Statistical integration for machine learning
“When the crystal structure is covered in the database, it’s a good fit,” Pope said, referring to the PNNL-grown flakes that are beginning to support the demonstration results.
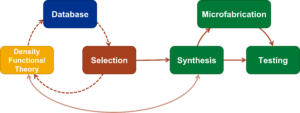
“The idea is really to create a large database of biological simulations so that we can use data analysis to understand these things,” Prange said. “The immediate benefit of this project is that we have covered enough different cases to effectively use machine learning.”
The open data, published in the journal “Data Science” of the Nature Publishing Group, provides researchers with a solid starting point to determine the relationship between the original structure and the corresponding elements. With this information, they can choose some things to study.
This project is an example of how we can use big computational data to conduct experimental research,” said CDI Chief Scientist Peter Sushko. “Projects like this provide important data for machine learning communities and can It is exciting to think about what needs to be understood next to make the connection of these things with atomic precision.
Source: Pacific Northwest National Laboratory